The playbook
Level up your sales strategy, management approach and tactics.
Featured posts
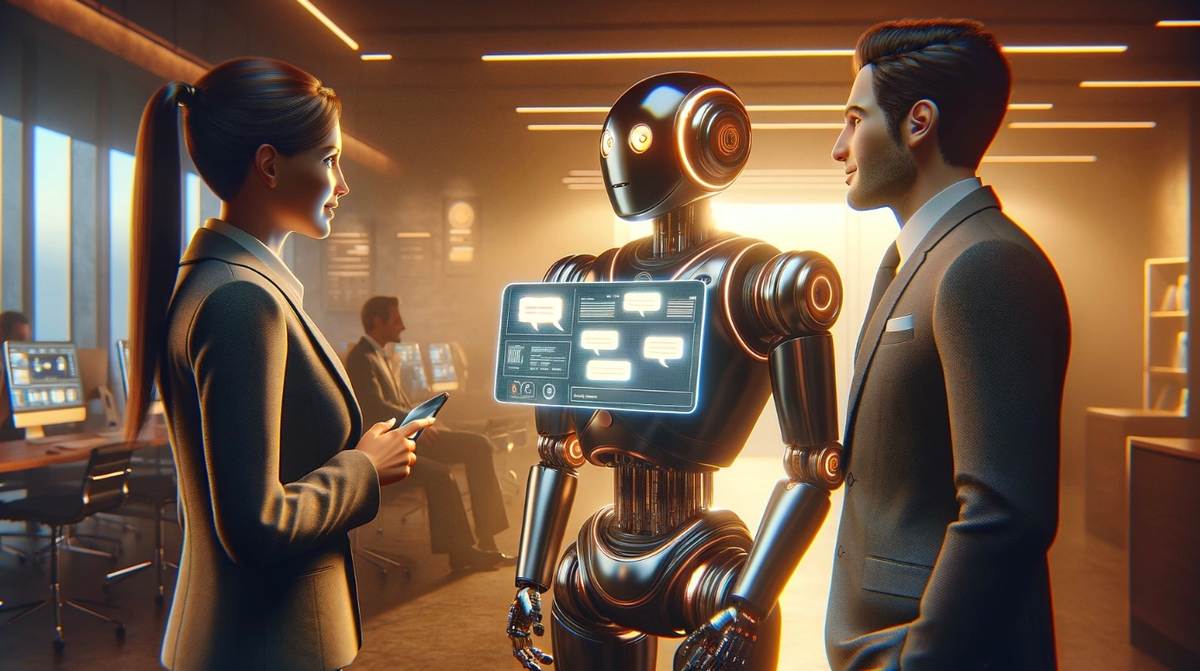
Sales management•10 mins
AI for Sales Managers: 3 Practical Ways to Use AI to Improve Team Outcomes
Nathan Clark|Oct 31, 2023
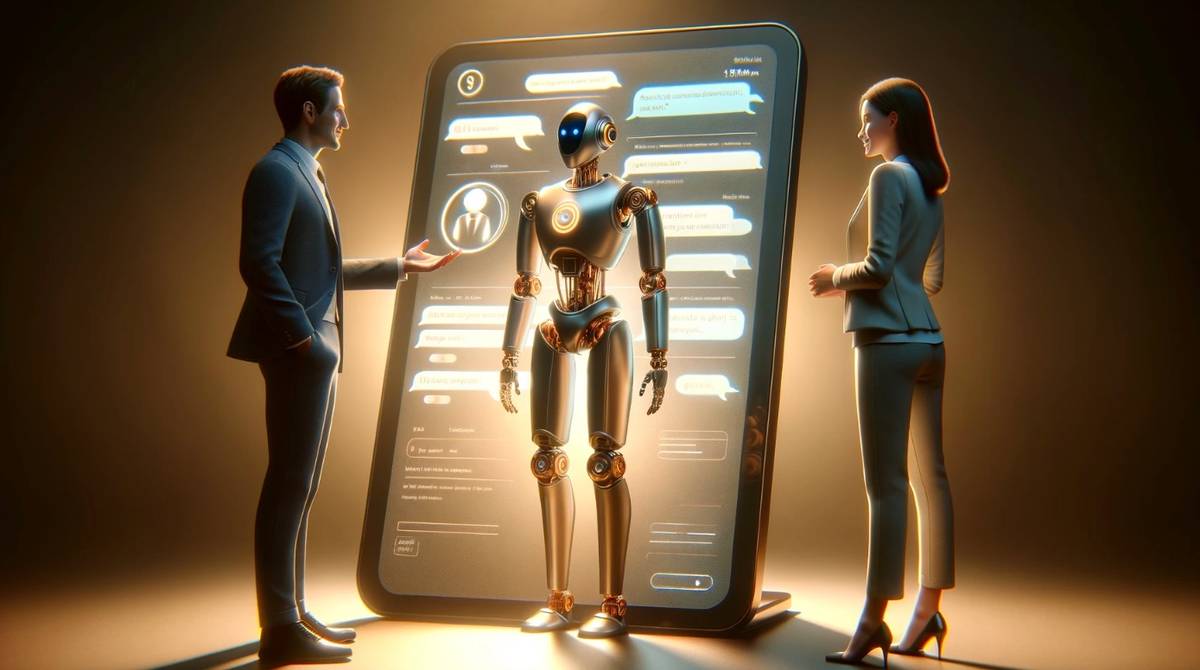
Sales enablement•14 mins
AI for Sales Reps: 3 Practical Ways to Increase Selling Time
Nathan Clark|Oct 16, 2023
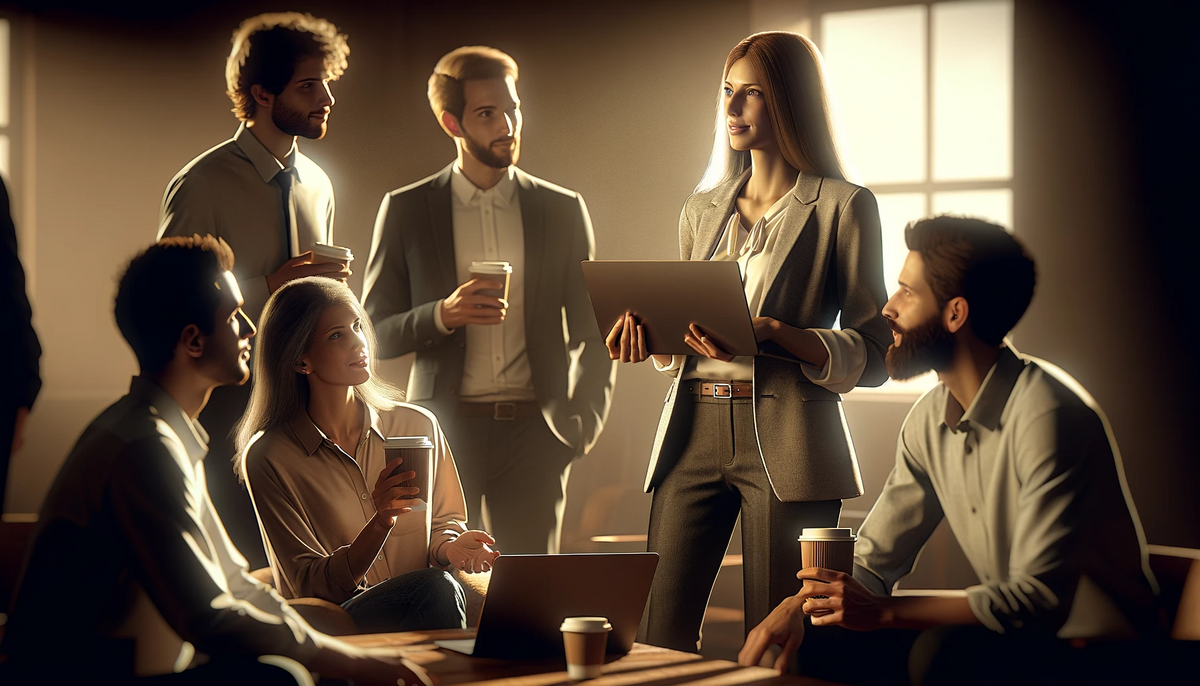
Sales enablement•19 mins
Mastering Sales Excellence: Strategies, Culture, and Tools
Brendan Connaughton|Dec 16, 2023
All posts
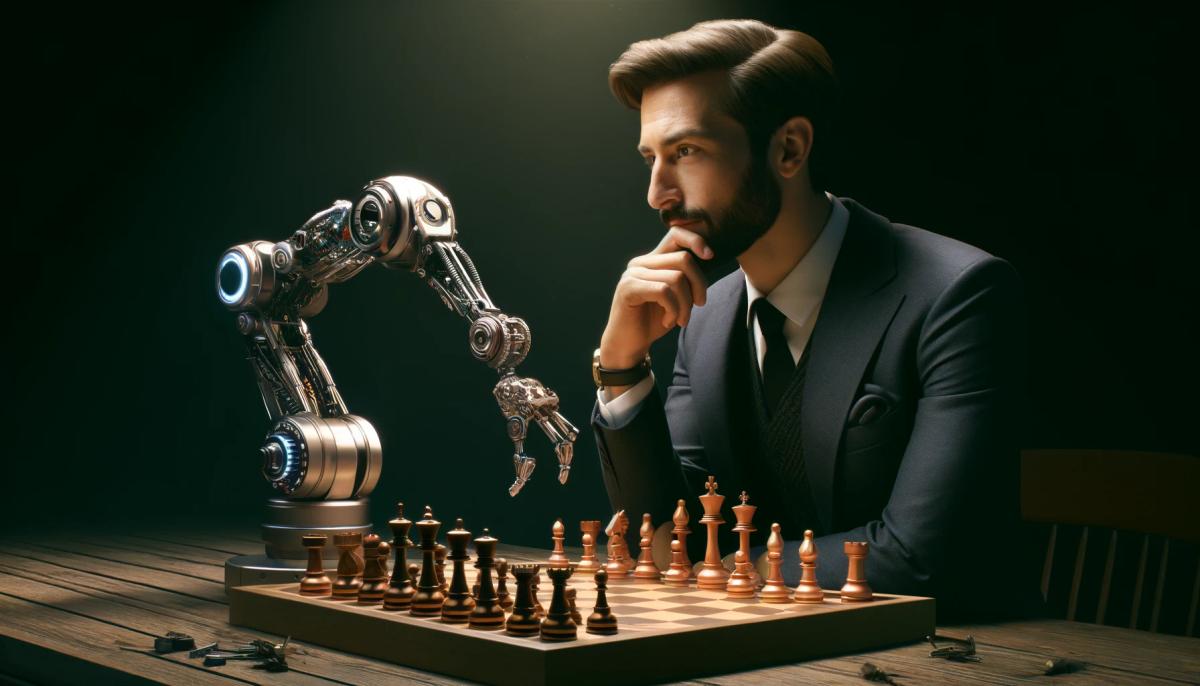
Sales techniques•29 mins
32 Sales Tactics You Should Use in 2024
Antonio Gabric|Apr 24, 2024
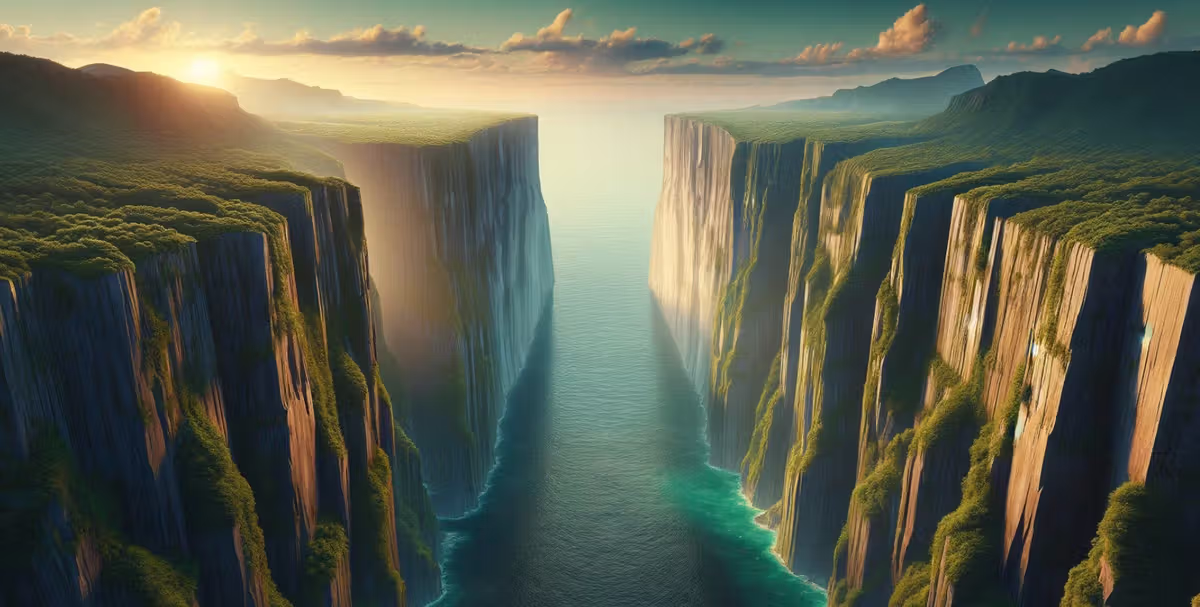
Sales techniques•18 mins
Gap Selling Methodology: Complete Framework for 2024
Apr 23, 2024
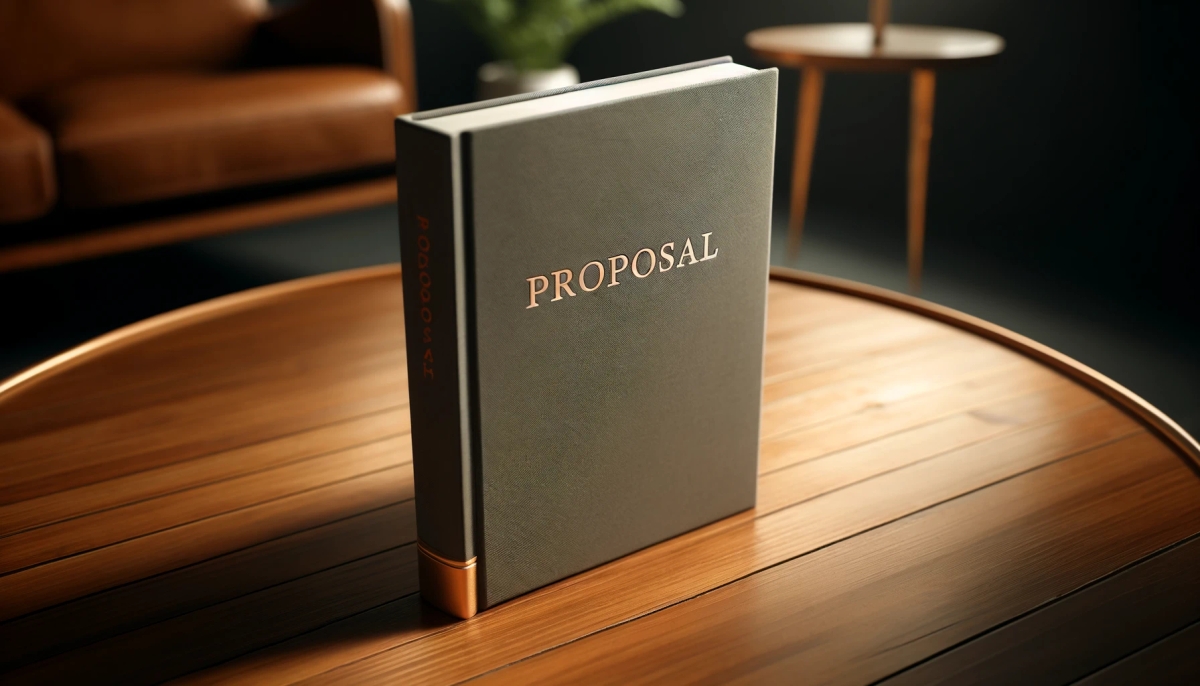
Sales enablement•9 mins
How Do You Structure A Proposal Cover Page
Marissa Taffer|Apr 20, 2024
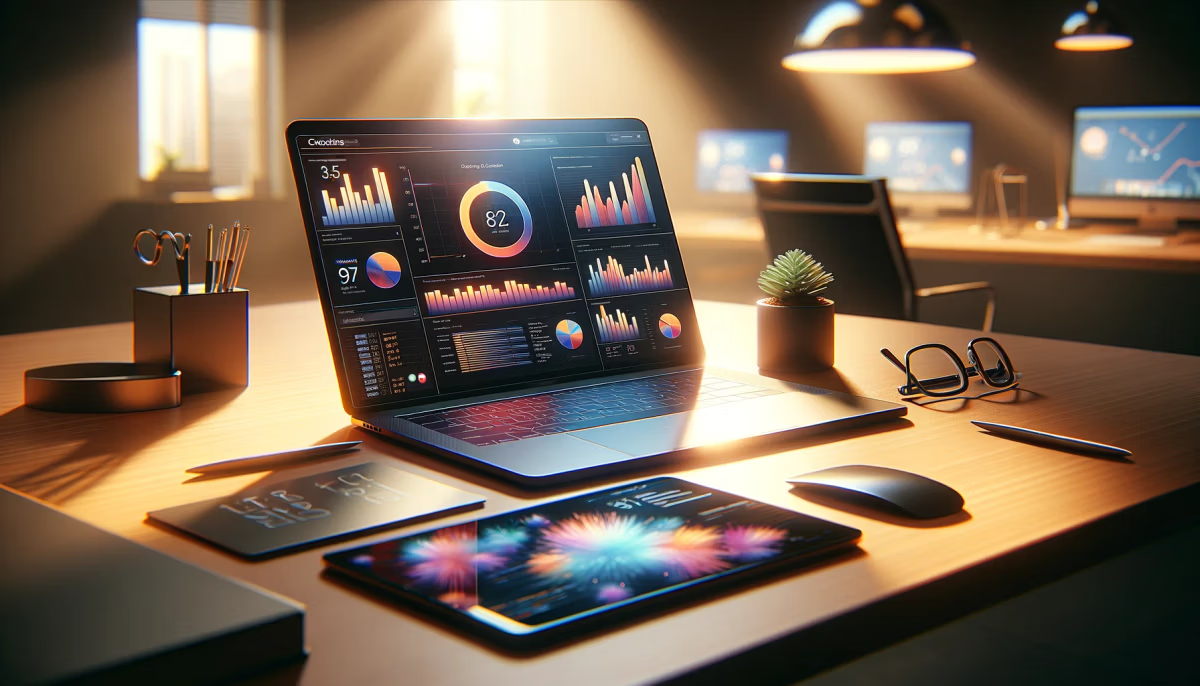
Marketing•16 mins
Effective Marketing Proposal Examples: Strategies to Captivate and Convert Clients
Marissa Taffer|Apr 10, 2024
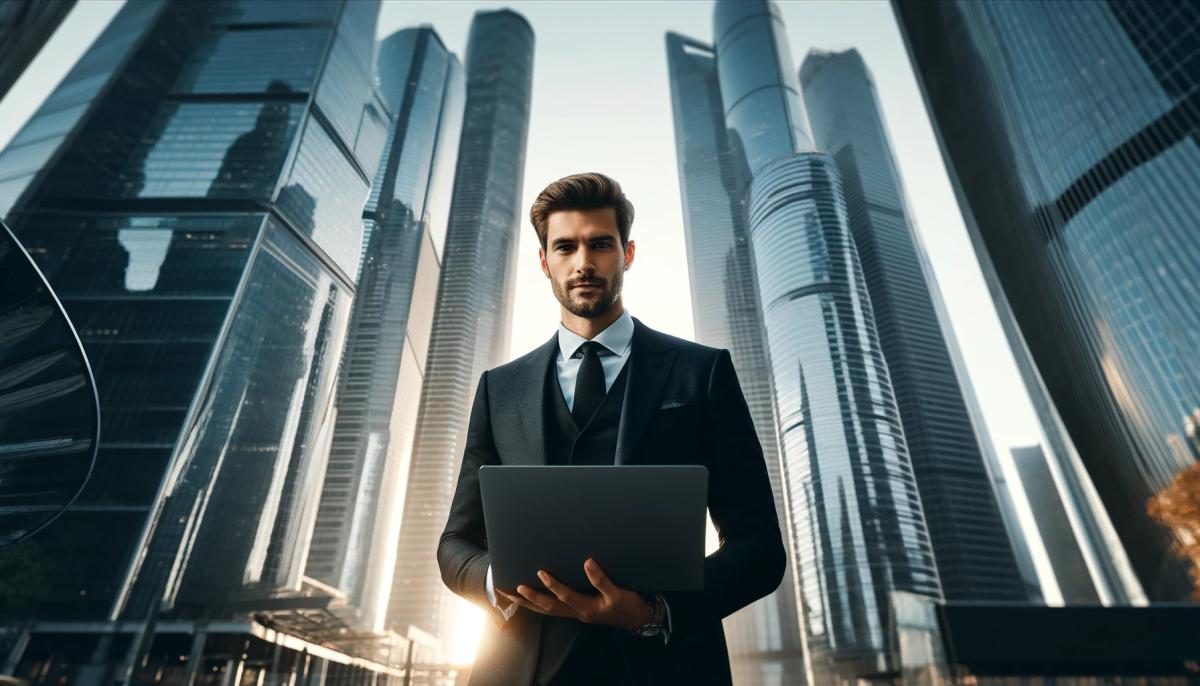
Sales techniques•6 mins
How to Write a High-Impact Commercial Insurance Proposal
Brendan Connaughton|Apr 4, 2024
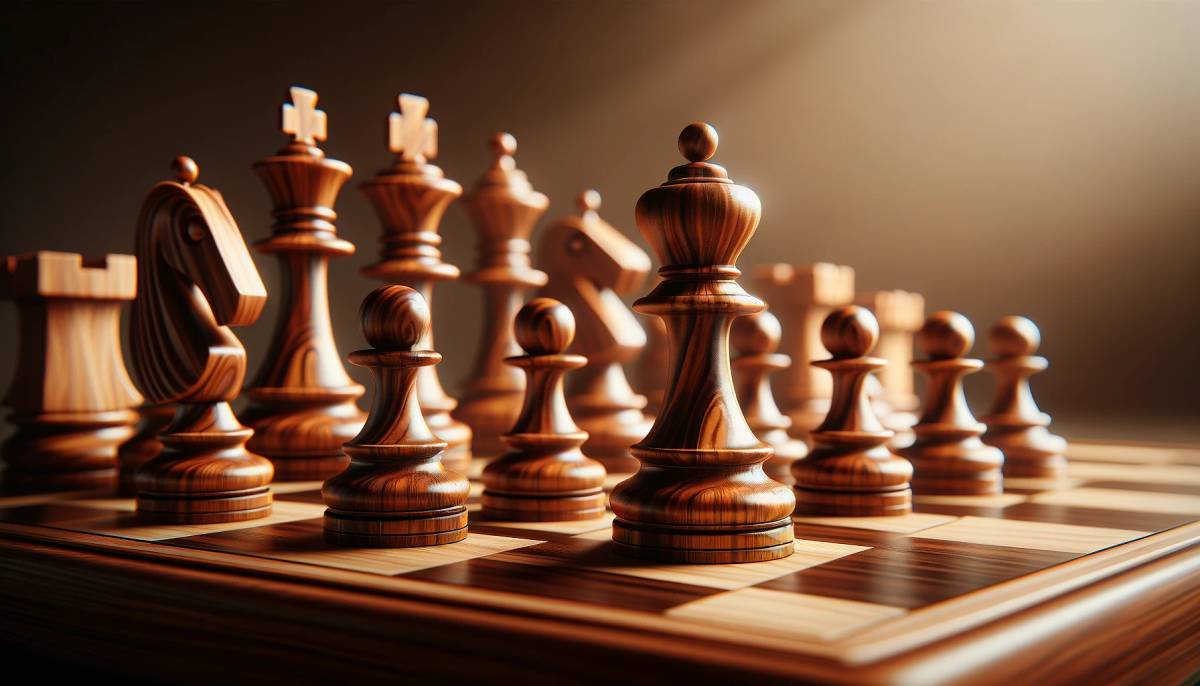
Sales management•19 mins
How To Build a SaaS Sales Strategy: 7 Key Considerations
Dan Lever|Apr 3, 2024

Sales techniques•10 mins
How to Write a Compelling Brand Collaboration Proposal
Dan Lever|Apr 2, 2024

Sales enablement•8 mins
MEDDIC vs. MEDDPICC: Which One Do You Use?
Marissa Taffer|Mar 29, 2024
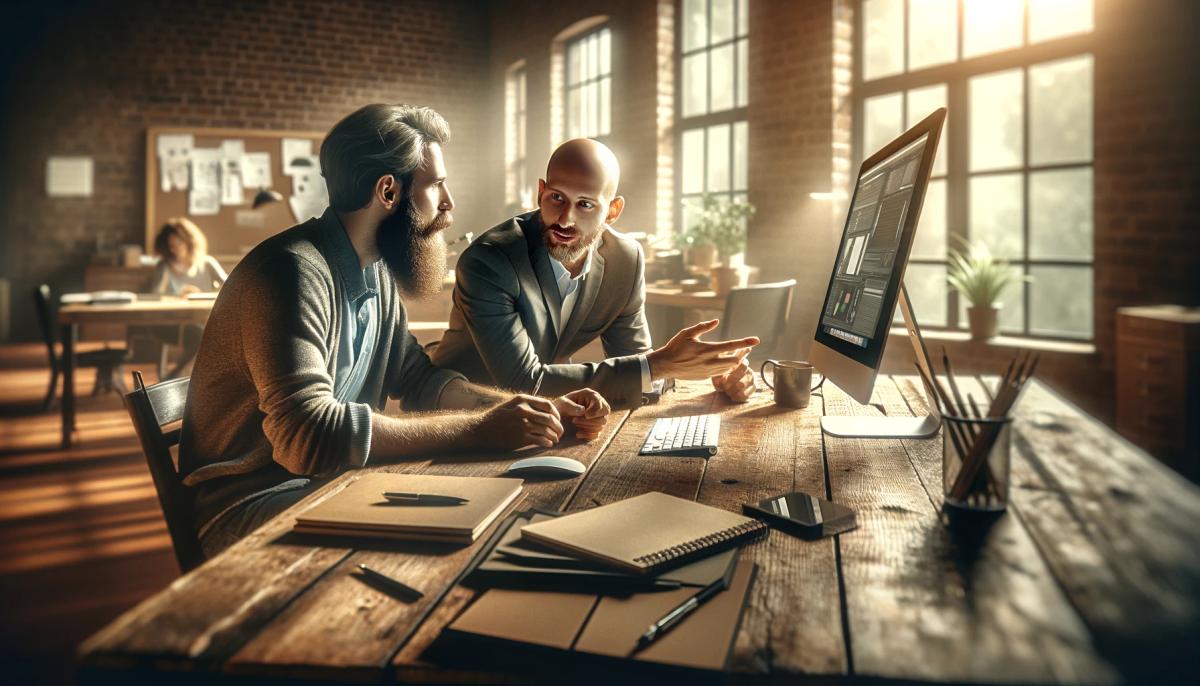
Sales enablement•7 mins
25 Essential BANT Questions to Qualify Leads Effectively
Marissa Taffer|Mar 28, 2024
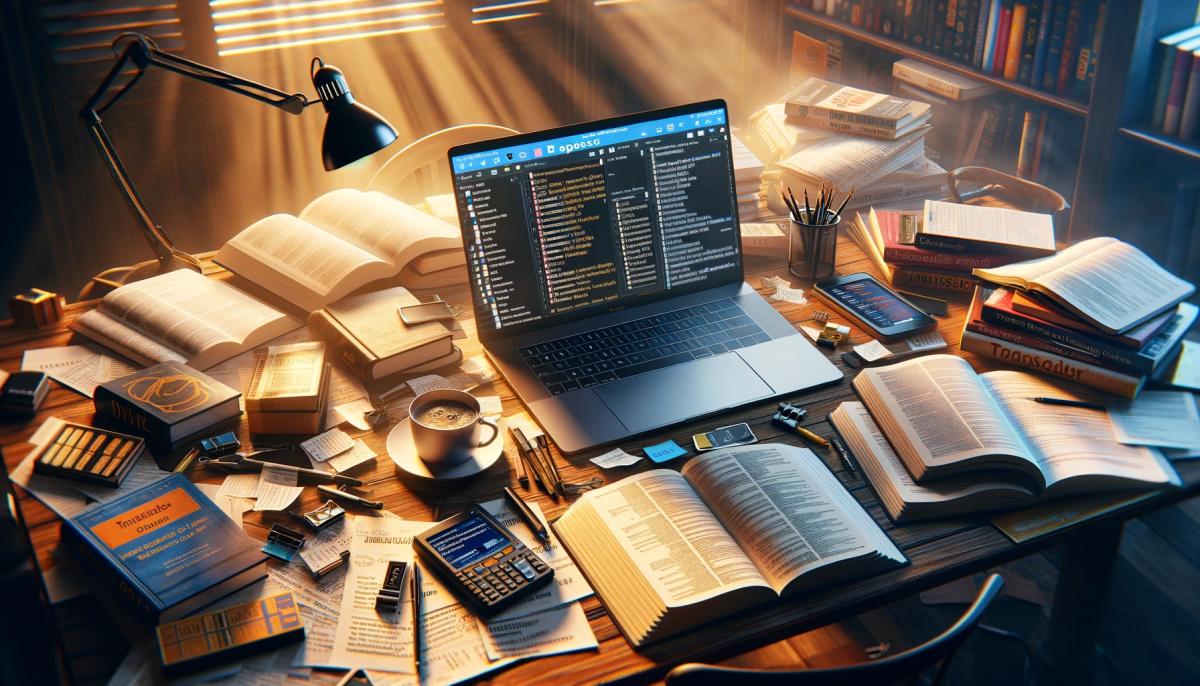
Sales management•11 mins
How to Write a Compelling Audit Proposal
Dan Lever|Mar 27, 2024
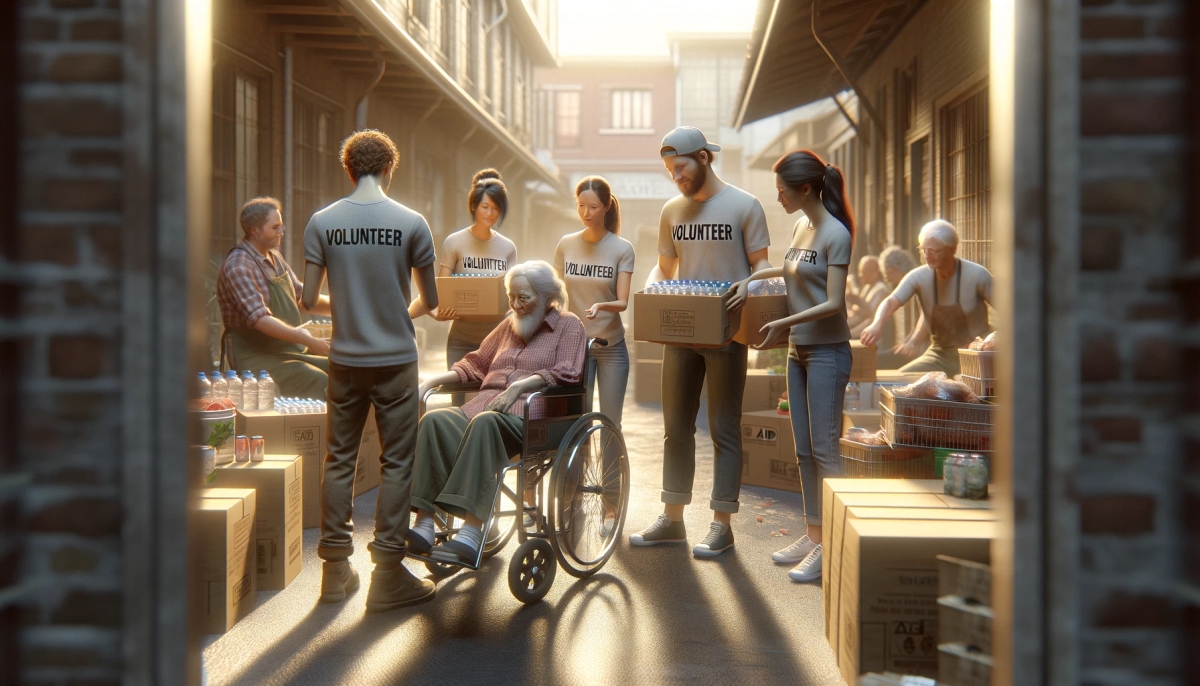
Product•11 mins
How to Write a Winning Fundraising Proposal
Brendan Connaughton|Mar 26, 2024
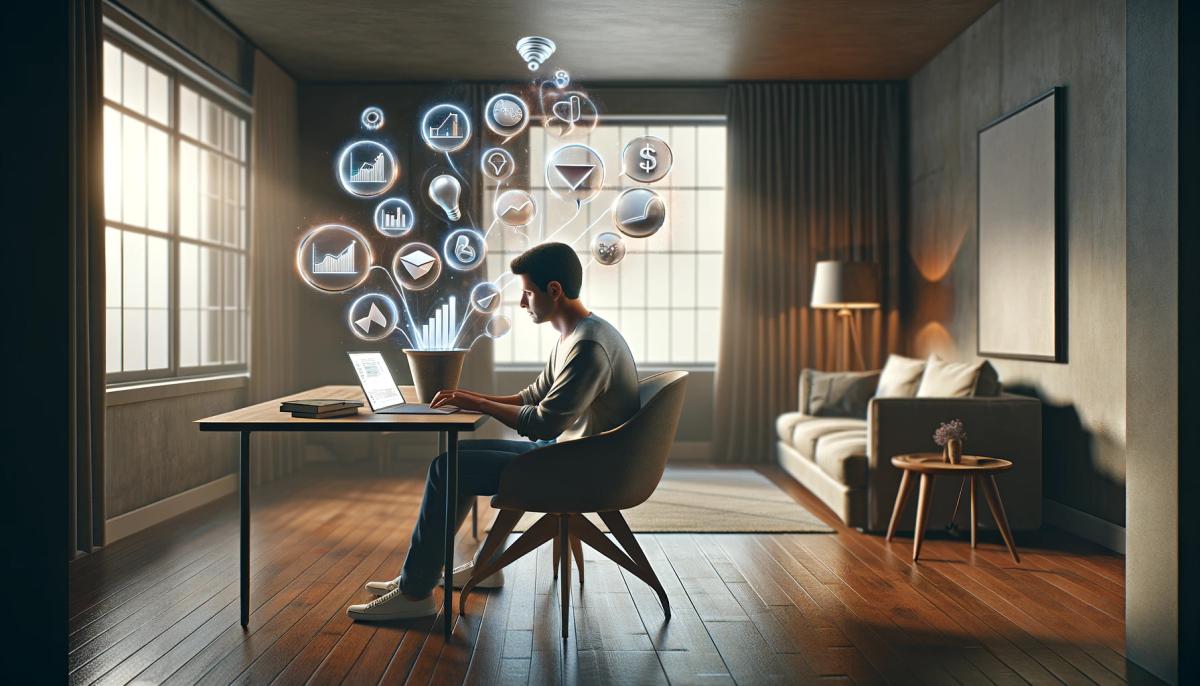
Sales management•11 mins
How to Write a Compelling CRM Proposal for 2024
Brendan Connaughton|Mar 22, 2024
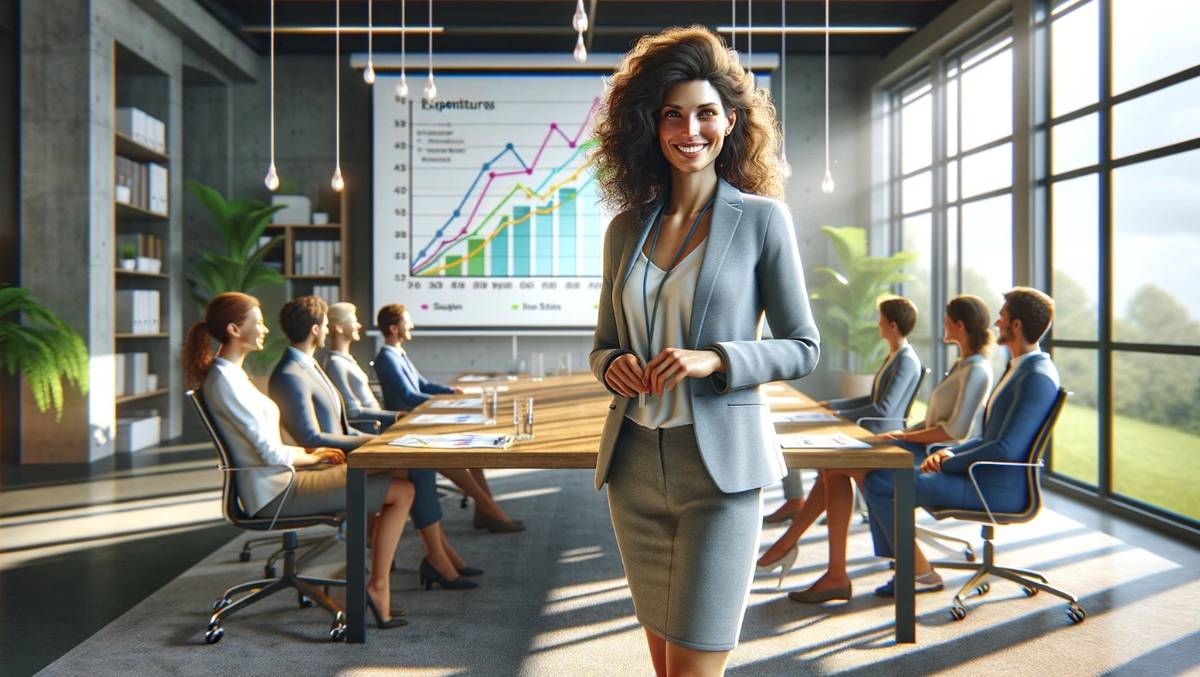
Sales techniques•21 mins
What Is Enterprise Sales? (Includes Key Tactics and Strategies)
Marissa Taffer|Mar 21, 2024
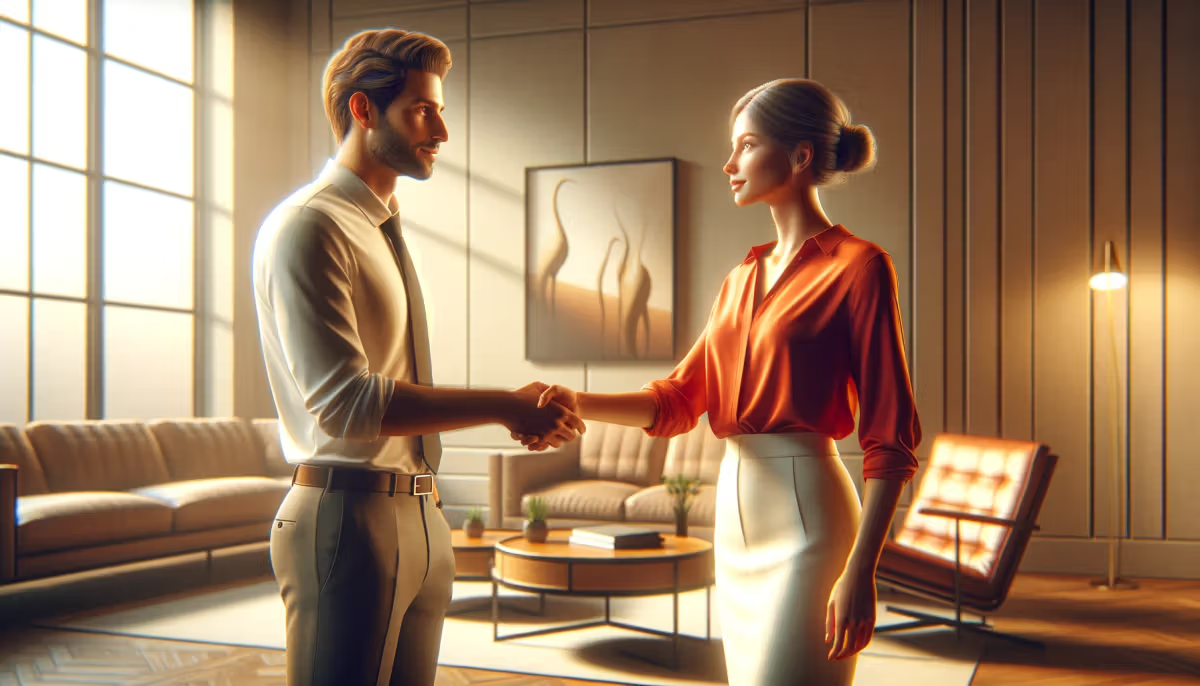
Marketing•13 mins
25 Essential MEDDIC Questions to Propel Deals Forward
Marissa Taffer|Mar 21, 2024
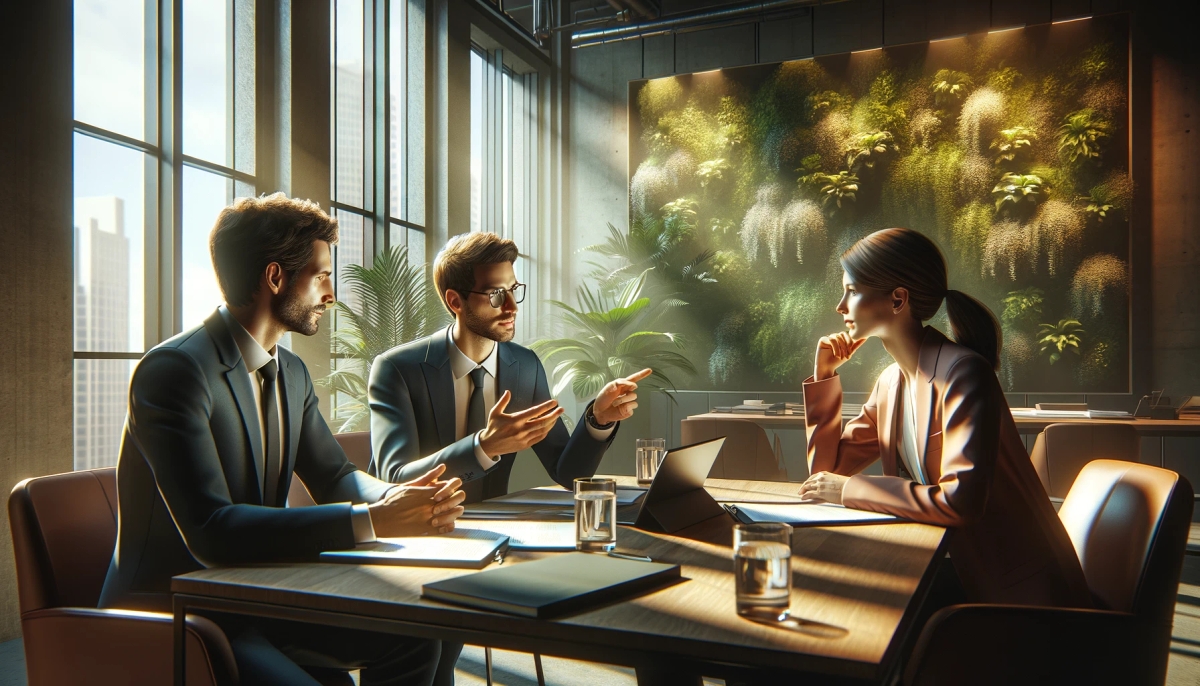
Sales techniques•7 mins
How to Write An Executive Coaching Proposal
Brendan Connaughton|Mar 21, 2024
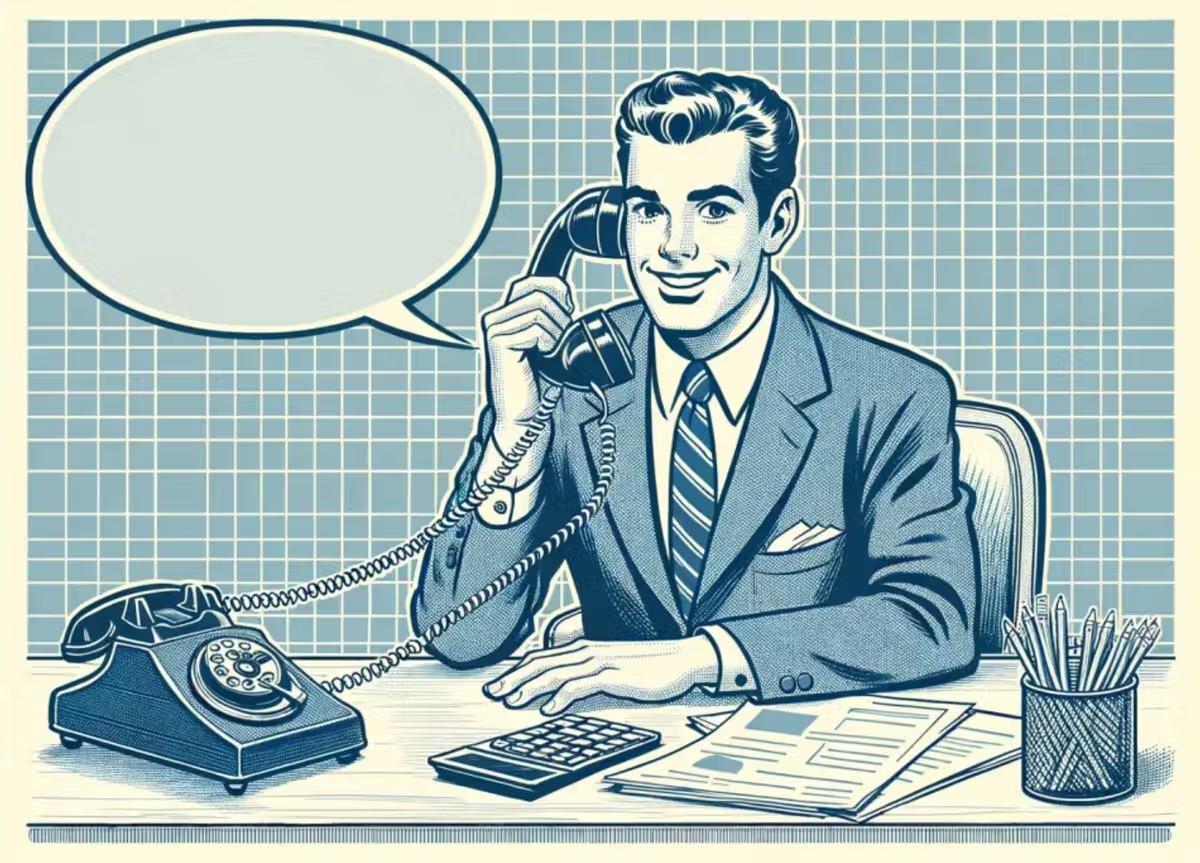
Sales techniques•8 mins
How To Make an Effective Sales Cold Call (+ Scripts)
Mar 18, 2024
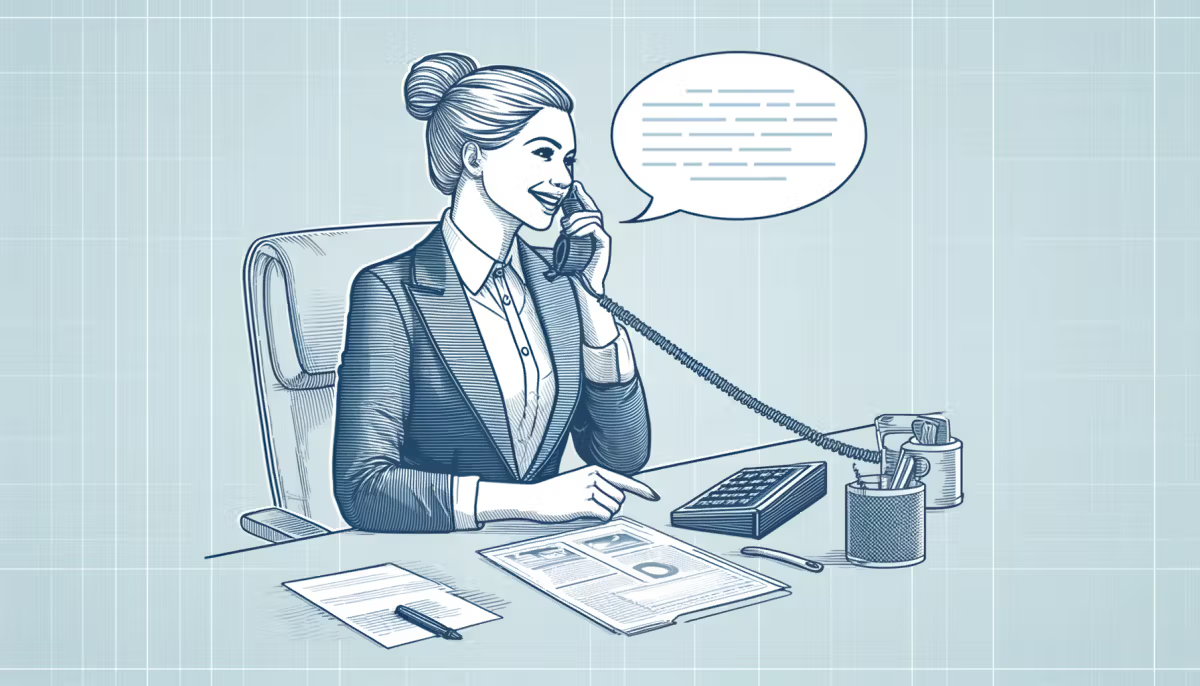
Sales techniques•5 mins
How To Get Past Any Gatekeeper in Sales (+ Scripts)
Mar 18, 2024
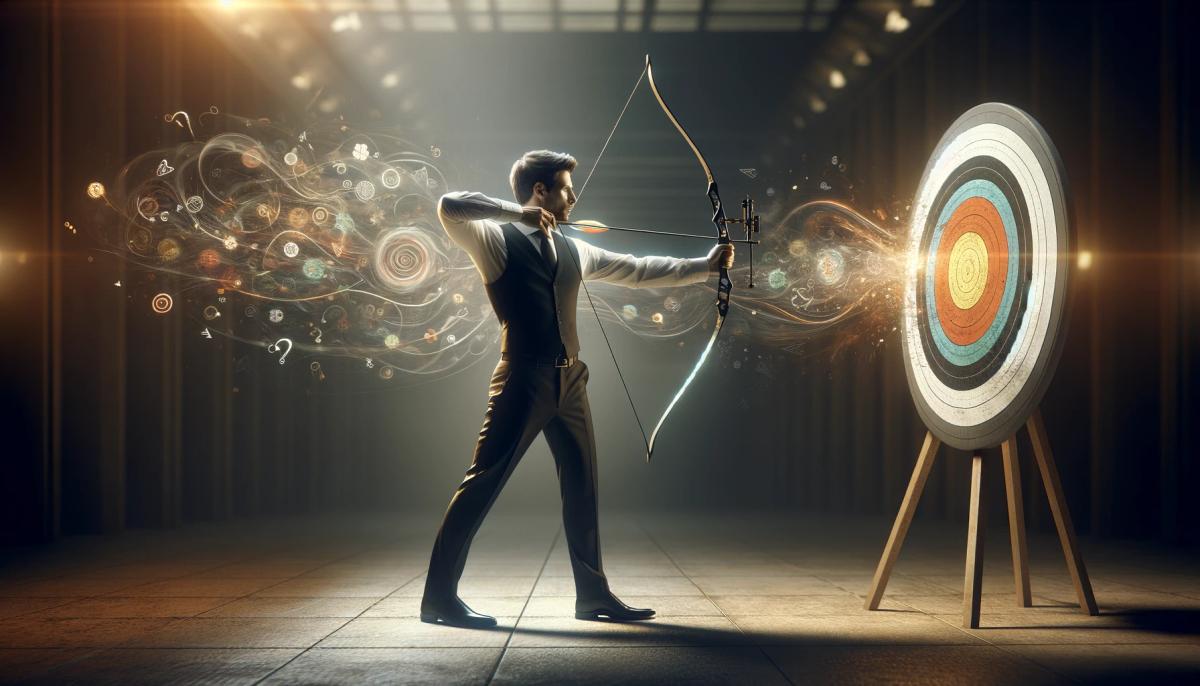
Sales techniques•9 mins
Sales ICPs: The Key to Better Targeting
Marissa Taffer|Mar 16, 2024